Project 9 - Integrating imaging, clinical and genetic data with machine learning to establish biomarkers for retinal diseases
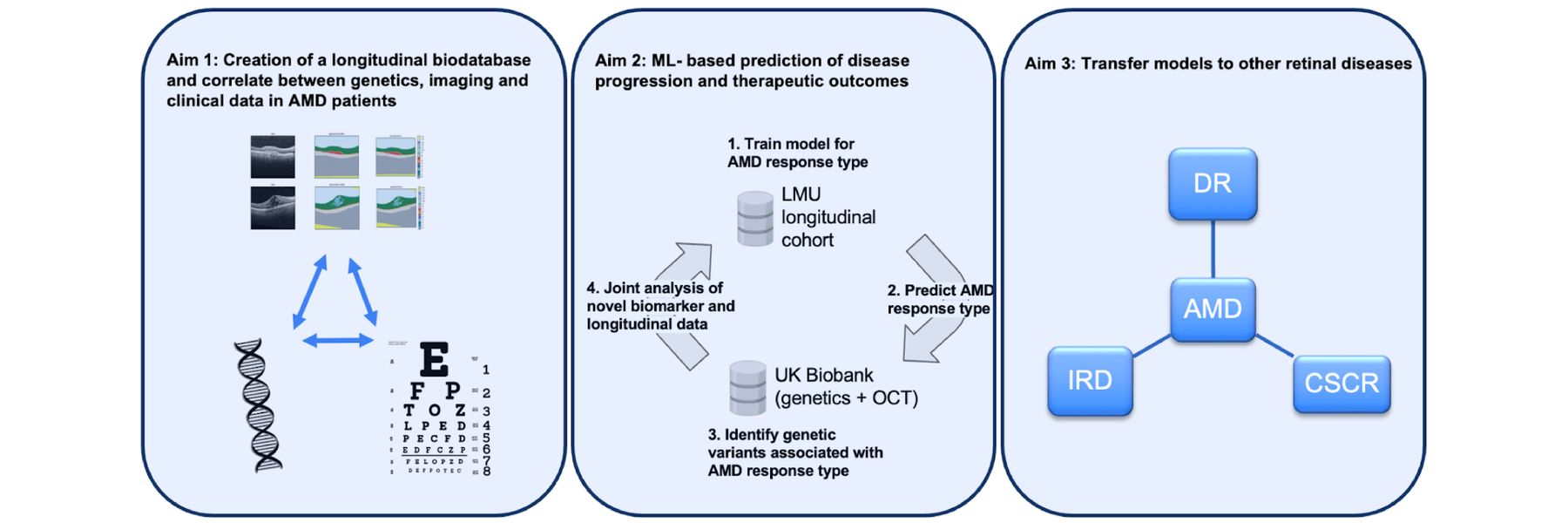
Retinal diseases, whether hereditary or acquired, often lead to a disturbance or destruction of retinal layers, which usually results in irreversible vision loss and, in the worst cases, legal blindness. Age-related neovascular macular degeneration is one of the leading causes of visual loss and blindness in the Western world. The pharmacological inhibition of the vascular endothelial growth factor (VEGF) as become the gold standard in treating neovascular AMD over the past two decades. Today, the therapeutic decisions are largely guided by biomarkers such as baseline visual acuity, choroidal neovascularization (CNV) lesion size and quantifiable features from optical coherence tomograph (OCT) imaging. However, the current therapeutic guidance has two main limitations: they do not fully utilize the breadth of information available in imaging data due to challenges associated with large scale image analysis, and they do not integrate genetic information, despite its increasing availability and potential to predict personalized outcomes.
Together with the Priglinger Lab, we aim to bridge these gaps by identifying connections between genetic factors, imaging data, and other clinical information in AMD patients through association studies and integrative machine learning models for longitudinal predictions. This project builds upon previous research by our groups, extending our current modeling approaches by incorporating patient genetic data. We intend to leverage artificial intelligence (AI), specifically machine learning (ML) and convolutional neural network (CNN) algorithms, which have shown immense promise in processing and analyzing vast amounts of complex medical data. This initiative is particularly crucial for common retinal diseases like AMD, which present large imaging datasets for machine learning and are likely to be among the first beneficiaries of this integration of biomedical imaging and genetics.